Creating parallel applications with the Julia programming language
Getting Parallel
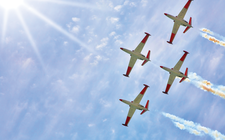
© Lead Image © Kavram, 123RF.com
Parallel processing is indispensable today – particularly in the field of natural sciences and engineering. Normal desktop users, however, can also benefit from higher performance through parallel execution with at least four calculation cores.
Programming tools such as MPI and OpenMP offer parallel processing features. It is easy to use these parallel language extensions, but using them efficiently is difficult because many algorithms cannot be rewritten for them. Languages such as Python and R also include parallel extensions, but these extensions were added on after the original language development and tend to be extremely slow when it comes to numerical calculations.
Many developers are looking for a language that is specifically designed with the intention of supporting parallel processing, and they want this parallel language to be easy to handle, with built-in features that facilitate parallelization and offer performance close to the blazing speed of C. A new language called Julia was developed to fill this niche (see the box titled "Julia Performance").
Hello Julia!
The current Julia version is available from GitHub [1]:
[...]
Buy this article as PDF
(incl. VAT)
Buy Linux Magazine
Subscribe to our Linux Newsletters
Find Linux and Open Source Jobs
Subscribe to our ADMIN Newsletters
Support Our Work
Linux Magazine content is made possible with support from readers like you. Please consider contributing when you’ve found an article to be beneficial.
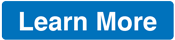
News
-
Fedora Continues 32-Bit Support
In a move that should come as a relief to some portions of the Linux community, Fedora will continue supporting 32-bit architecture.
-
Linux Kernel 6.17 Drops bcachefs
After a clash over some late fixes and disagreements between bcachefs's lead developer and Linus Torvalds, bachefs is out.
-
ONLYOFFICE v9 Embraces AI
Like nearly all office suites on the market (except LibreOffice), ONLYOFFICE has decided to go the AI route.
-
Two Local Privilege Escalation Flaws Discovered in Linux
Qualys researchers have discovered two local privilege escalation vulnerabilities that allow hackers to gain root privileges on major Linux distributions.
-
New TUXEDO InfinityBook Pro Powered by AMD Ryzen AI 300
The TUXEDO InfinityBook Pro 14 Gen10 offers serious power that is ready for your business, development, or entertainment needs.
-
Danish Ministry of Digital Affairs Transitions to Linux
Another major organization has decided to kick Microsoft Windows and Office to the curb in favor of Linux.
-
Linux Mint 20 Reaches EOL
With Linux Mint 20 at its end of life, the time has arrived to upgrade to Linux Mint 22.
-
TuxCare Announces Support for AlmaLinux 9.2
Thanks to TuxCare, AlmaLinux 9.2 (and soon version 9.6) now enjoys years of ongoing patching and compliance.
-
Go-Based Botnet Attacking IoT Devices
Using an SSH credential brute-force attack, the Go-based PumaBot is exploiting IoT devices everywhere.
-
Plasma 6.5 Promises Better Memory Optimization
With the stable Plasma 6.4 on the horizon, KDE has a few new tricks up its sleeve for Plasma 6.5.