A tour of some important data science techniques
Method in the Madness
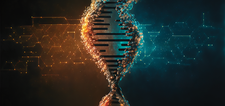
Data science is all about gaining insights from mountains of data. We tour some important tools for the trade.
Data is the new oil, and data science is the new refinery. Increasing volumes of data are being collected, by websites, retail chains, and heavy industry, and that data is available to data scientists. Their task is to gain new insights from this data while automating processes and helping people make decisions [1]. The details for how they coax real, usable knowledge from these mountains of data can vary greatly depending on the business and the nature of the information. But many of the mathematical tools they use are quite independent of the data type. This article introduces you to some of the methods data scientists use to squeeze insights from a sea of numbers.
More than Just Modeling
The term data scientist evokes associations with math nerds, but data science consists of far more than building and optimizing models. First and foremost, it involves understanding a problem and its context.
For example, imagine a bank wants to use an algorithm to predict the probability that a borrower will be able to repay a loan. A data scientist will first want to understand how lending has worked so far and what data has been collected in this field – as well as whether that data is actually available – with a view to data protection requirements. In addition, data scientists need to be able to communicate their findings. Storytelling is more useful than presenting infinite rows of numbers, because the audience is likely to be made up of non-mathematicians. The need to clearly explain the findings frequently presents a challenge for less extroverted data scientists.
[...]
Buy this article as PDF
(incl. VAT)
Buy Linux Magazine
Subscribe to our Linux Newsletters
Find Linux and Open Source Jobs
Subscribe to our ADMIN Newsletters
Support Our Work
Linux Magazine content is made possible with support from readers like you. Please consider contributing when you’ve found an article to be beneficial.
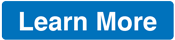
News
-
Endless OS 6 has Arrived
After more than a year since the last update, the latest release of Endless OS is now available for general usage.
-
Fedora Asahi 40 Remix Available for Macs with Apple Silicon
If you've been anticipating KDE's Plasma 6 for your Apple Silicon-powered Mac, then you're in luck.
-
Red Hat Adds New Deployment Option for Enterprise Linux Platforms
Red Hat has re-imagined enterprise Linux for an AI future with Image Mode.
-
OSJH and LPI Release 2024 Open Source Pros Job Survey Results
See what open source professionals look for in a new role.
-
Proton 9.0-1 Released to Improve Gaming with Steam
The latest release of Proton 9 adds several improvements and fixes an issue that has been problematic for Linux users.
-
So Long Neofetch and Thanks for the Info
Today is a day that every Linux user who enjoys bragging about their system(s) will mourn, as Neofetch has come to an end.
-
Ubuntu 24.04 Comes with a “Flaw"
If you're thinking you might want to upgrade from your current Ubuntu release to the latest, there's something you might want to consider before doing so.
-
Canonical Releases Ubuntu 24.04
After a brief pause because of the XZ vulnerability, Ubuntu 24.04 is now available for install.
-
Linux Servers Targeted by Akira Ransomware
A group of bad actors who have already extorted $42 million have their sights set on the Linux platform.
-
TUXEDO Computers Unveils Linux Laptop Featuring AMD Ryzen CPU
This latest release is the first laptop to include the new CPU from Ryzen and Linux preinstalled.